Nutribullet’s new Flip incorporates a blender into an insulated stainless steel tumbler that looks no larger than a typical travel mug. If you don’t have enough time in the morning to blend your breakfast, the rechargeable Flip works anywhere you don’t mind making a bit of noise. (Maybe skip the crowded subway.)
Business
FPCCI hosts conference to address IPP issues, high electricity costs
Former caretaker Prime Minister Anwaar-ul-Haq Kakar says the problem of IPPs is under discussion in the Senate; the rights of the people have been fighting for the small battles of the people before
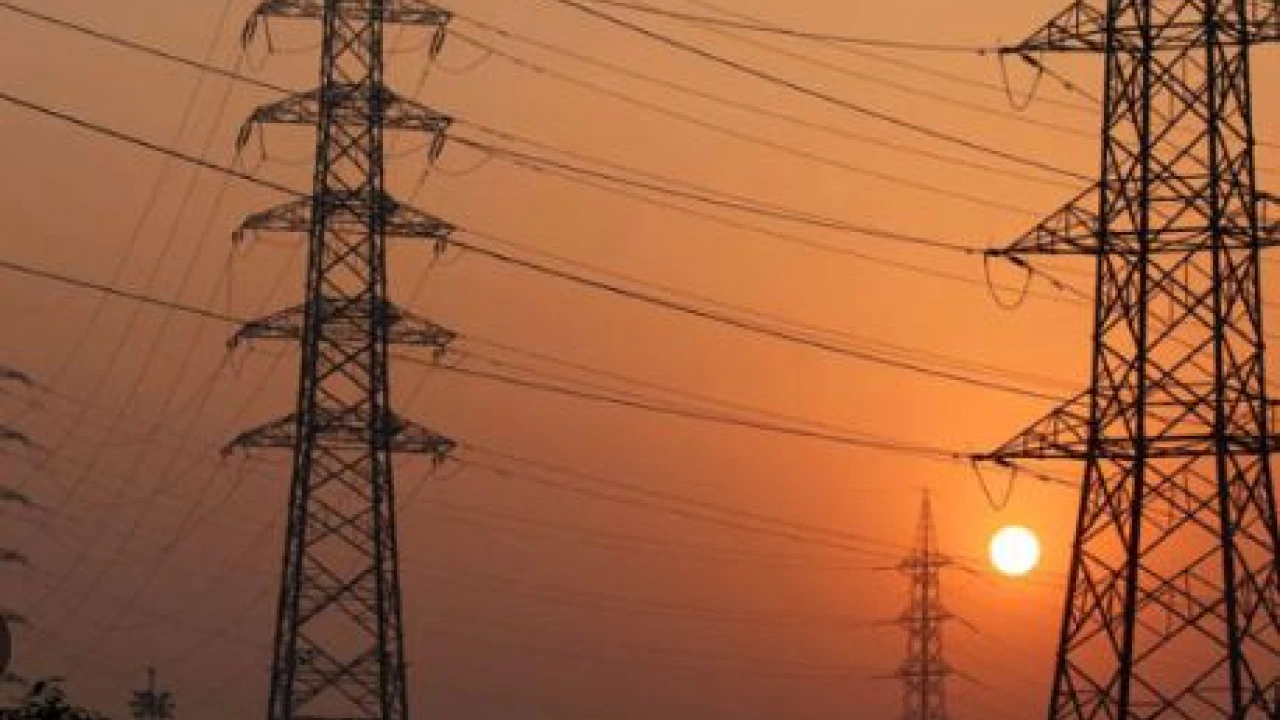
Lahore: The Federation of Pakistan Chambers of Commerce and Industry (FPCCI) “Businessmen Conference” on Independent Power Producers (IPPs) was held at FPCCI Regional Office Lahore on Friday.
Addressing the FPCCI “Businessmen Conference,” Former caretaker Prime Minister Anwaar-ul-Haq Kakar said that the issue of IPPs is 24 million people, including industry. The problem of IPPs is under discussion in the Senate; the rights of the people have been fighting for the small battles of the people before. Astronomy sitting on social media is spreading frustration about the country; the future of the country is bright. IPPs are not scoring points by raising the issue. We will resolve the problem through dialogue.
Former Caretaker Federal Minister for Commerce, Industries, Investment & Interior and FPCCI Economic Policy Business Development Think Tank Chairman Dr Gohar Ejaz said that the negligence of the government agencies of IPPs was being put on the public. A forensic audit of IPPs should be conducted. The whole of Pakistan is saying with a voice that has been affected by the IPPs that we have to find a solution to terminate the deal with IPPs in every case. We never want our country to go into crisis. There will be a policy that Pakistan will move forward. There is electricity, but the problem is the cost of electricity. How will we fight the world for expensive electricity?
Atif Ikram Sheikh, President FPCCI and Patron-in-Chief United Business Group (UBG) and Former Caretaker Provincial Minister for Industries, Commerce and Energy and Patron in Chief United Business Group (UBG) SM Tanveer announced that, on behalf of the entire business, industry, and trade community of Pakistan, FPCCI would plead the case of unbearable capacity charges of IPPs to the apex forums of the Supreme Court of Pakistan and Special Investment Facilitation Council (SIFC)-as the last resort to save the economy and the people of Pakistan.
They stressed that the problem would also be explained and a solution given. The business community has said industries are shutting down due to expensive electricity and excessive markups.
They further said that it is impossible to do business at this electricity rate. Anwaar-ul-Haq Kakarhas has been elected president of the FPCCI Economic Policy Business Development Think Tank. Electricity is so expensive. The electricity bill is unbearable. Due to incorrect contracts, capsat charges have to be paid; electricity prices are the biggest problem in Pakistan right now. Annual Rs 2,000 billion is being removed from the pockets of 24 crore people regarding capacity charges, and 25 % of the industry has been closed. Such IPPs cannot run the country.
Technology
There’s a blender hidden inside this insulated travel cup
The Nutribullet Flip can blend frozen ingredients for up to seven minutes on a full charge and keep smoothies cold for up to 24 hours.
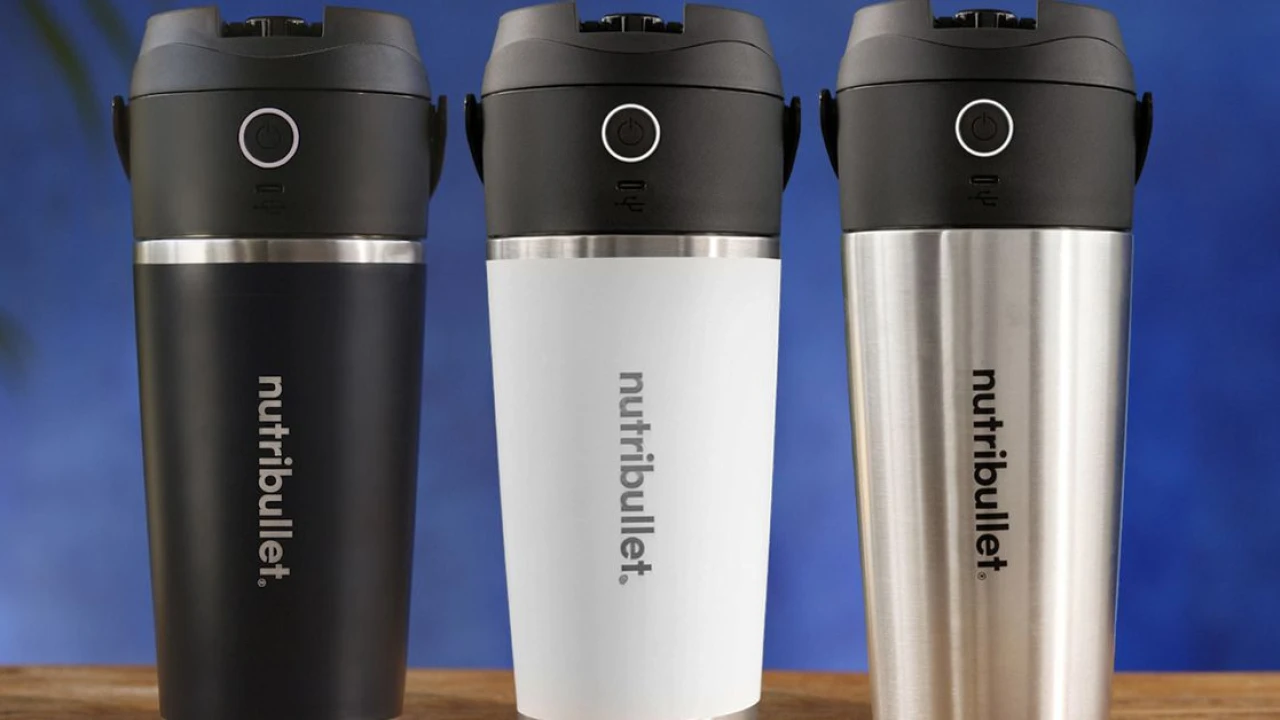
The $99.99 Flip isn’t Nutribullet’s first single-serve blender, nor is it the company’s first rechargeable one. What sets it apart from past offerings is that the blender part — including blades, an 11.1-volt electric motor, and a rechargeable battery — are all incorporated into the Flip’s lid, instead of into a removable base that gets left behind. We’ve seen that idea before in the powerful Cruz BlenderCap, which costs $149.
On a full charge, the Nutribullet Flip can run a 30-second blend cycle over 14 times before it needs to be plugged back in via a USB-C charging cable. Seven minutes isn’t a lot of time but should be sufficient for blending up a drinkable breakfast or maybe lunch later in the day. The Cruz, meanwhile, gave us a small shake each day for a week.
You can fill the 20-ounce tumbler with whatever ingredients you want. Nutribullet claims the Flip’s motor is powerful enough to blend ice, fresh ingredients, and “most” frozen fruits. But it also warns against using heated ingredients, as they can cause pressure to build up inside that could result in the lid forcefully opening or completely separating from the tumbler.
When filled, the lid is then attached to the insulated tumbler, and the whole thing is inverted before blending so the contents fall onto the chopping blades. It’s also worth pointing out that the Flip weighs 3.45 pounds on its own. A 20-ounce Yeti mug, for comparison, is just over a pound. Adding ice and frozen fruit is going to give you a real workout when carrying this around.
Once complete, the lid can be left on and used for pouring or drinking. The best feature is that when the Flip is empty it can be easily cleaned by filling it with soap and water and then running a single blend cycle. What you don’t want to do is submerge the Flip or stick it in the dishwasher, as that USB-C port is not waterproof.
Technology
How the Supreme Court’s Chevron ruling could doom net neutrality
Without Chevron deference, the FCC might not be able to protect net neutrality for long.
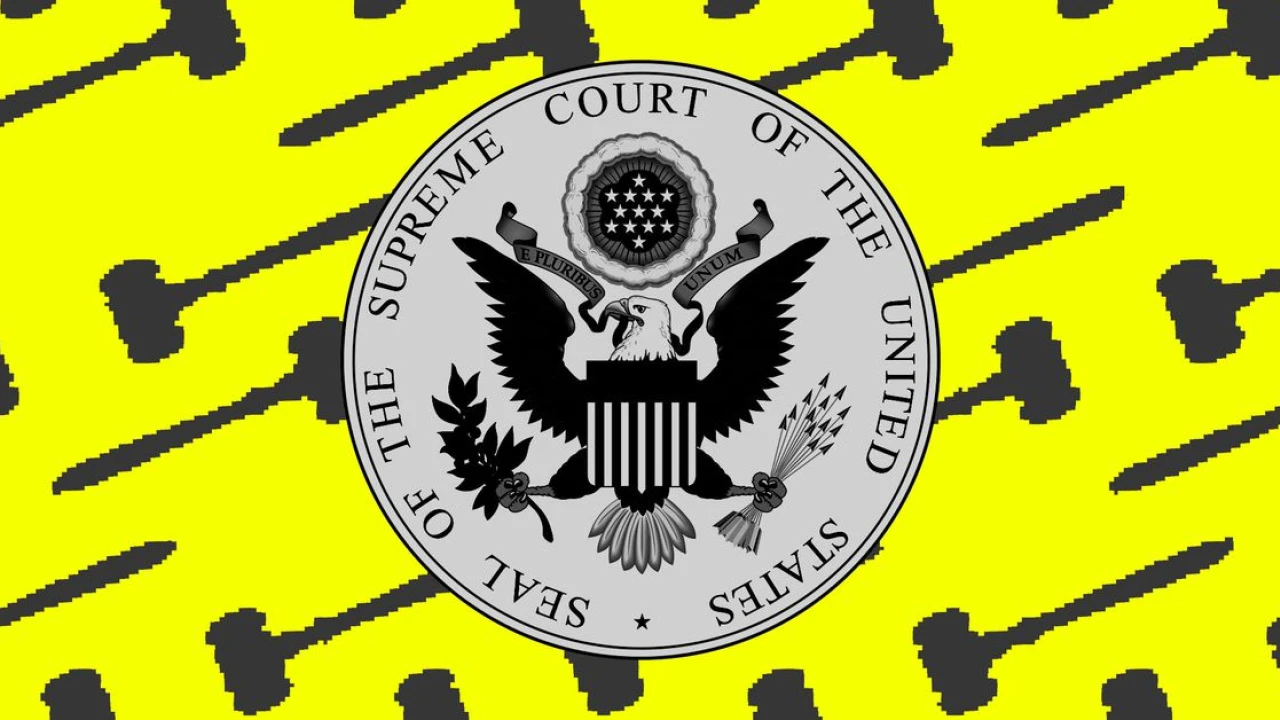
On today’s episode of Decoder, we’re talking about the Supreme Court’s decision to overturn Chevron deference and what it means for the future of the internet.
I’ve been covering the fight over net neutrality for almost my entire career as a tech reporter — over 15 years now. The idea is that internet service providers shouldn’t be able to discriminate between what services you access on the internet: AT&T shouldn’t be able to slow down X and speed up Threads, and Verizon shouldn’t be able to block Zoom because it owns BlueJeans.
It’s pretty basic stuff, and after all this time, the Biden administration’s Federal Communications Commission once again made it the law. But big telecom companies truly hate the idea that anyone would regulate their networks, and they once again filed a lawsuit over it. Until very recently, everyone sort of understood the arguments in a case like that and how they would go — like I said, I’ve been covering this for 15 years now. It felt familiar.
But then, everything changed. In late June, the Supreme Court issued a bombshell opinion in a case called Loper Bright Enterprises v. Raimondo, which overturned something called Chevron deference — the idea that courts should generally defer to agencies like the FCC when it comes to interpreting ambiguous parts of the law.
That means the net neutrality lawsuit is suddenly very different. It’s now about whether the court thinks the internet should be neutral, not the FCC. And wouldn’t you know it, the Sixth Circuit immediately halted the FCC’s new net neutrality order and asked for briefs on how the Loper Bright ruling would change the case.
I invited Verge editor Sarah Jeong on the show to talk about all of this with me, and you’ll really hear us get into it. I mean, for the last 40 years, judges have basically deferred to federal agencies when it comes to the details of interpreting law because the agencies employ experts and have really deep subject area knowledge.
But now, judges will be empowered to make their own interpretations and throw out old ones. And with a dysfunctional Congress that can barely pass any laws as it is, the Supreme Court’s decision in Loper Bright is a major power grab for the judiciary over the other two branches of government.
It’s a very big deal, with some far-reaching consequences for basically everything, including the environment, labor law, and all manner of regulation. Here at The Verge, our policy team has been tracking this outcome for a long time, so I wanted to have Sarah come on the show to break down how we got here and what it means for the future.
If that sounds really chaotic and unstable to you, well… you’re not wrong, and you’ll hear us discuss that toward the end, when we did our best to put this state of affairs into context and try to figure out what happens next.
-
World 2 days ago
Nepali Airlines plane skids, crashes during take-off, 18 killed
-
Weather 2 days ago
Weather turns pleasant after rains in Lahore, other cities
-
Regional 2 days ago
Rana Sanaullah lauds UNESCO's role in sports education
-
Weather 2 days ago
Rain likely at isolated places; humid weather to continue: PMD
-
Pakistan 2 days ago
Nearly 50,000 Pakistani pilgrims go missing in Iraq: Minister
-
Business 13 hours ago
Gold price up by Rs1,000 per tola in Pakistan
-
Pakistan 2 days ago
Nawaz, Zardari standing on wrong side of history: Fawad
-
World 2 days ago
Kamala leads Trump 44pc to 42pc in US presidential race